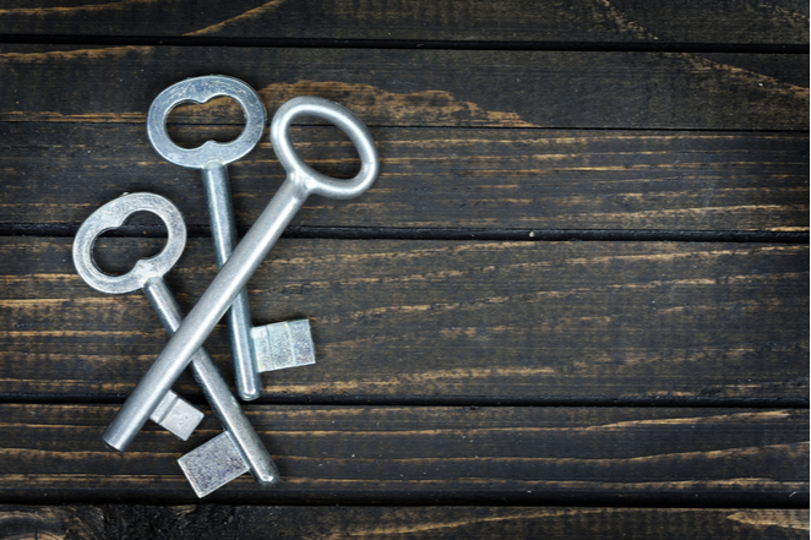
Shopper Research: 3 reasons why asking for the Key Takeouts may be wrong
We recently delivered a huge Shopper Intelligence project for a client where, I calculated, the data set created approached nearly 500,000 data points at the first “cut”. This wasn’t some Leviathon developed based on traffic measurement or social media. This is just what we usually do: post-shop interviews using a 10-15 minute questionnaire. But if you take a typical questionnaire with all the question options, you multiply it by several categories and a number of different retailers, add in the ability to cut it by segment, brand, customer type and so on, and very soon you have a LOT of different numbers.
Any of us who have done lots of quantitative studies will recognize the challenge. Turning that huge amount of information into “insights”. And yes, despite our prompting not to, typically a client will ask:
“So, what are the main takeouts?”.
A sensible question at one level (you need to show a quick ROI on your research budget, correct?). But it’s also potentially a very dangerous one.
Dangerous for three reasons.
First, this assumes the researchers are best placed to determine what “main” should be in the context of takeouts.
Second, that this is a quick task (particularly if this is being asked for as the first deliverable).
And thirdly, that this is a point in time, static task. That there are in fact a pre-determined set of takeouts inherent in the data that can be found processed, job done, move on.
Let’s take each in turn…
1. Are researchers best placed?
Well in some respects, yes. They are well placed. They are trained to do this. They are comfortable with lots of data. They are good with the tools (we have our own analytical software system and our people are VERY fast on it) and probably have spent the most time thinking about the nitty-gritty of the work compared to anyone else (i.e. planning it, executing it etc.).
But there’s one problem.
Inevitably they don’t know the category, the market or the client’s business as well as others will. So, as they look through the data often they don’t have anything to match up with in terms of business needs/concerns to filter what they are looking at. At best they may just go for the obvious learnings. Or at worst actively but inadvertently miss out on things a more informed viewer would think important.
True insights come from melding various sources of information, some of which are just part and parcel of living in a business every day, year-round. Furthermore, access to and knowledge of past work and other studies could be crucial. It’s rare these are shared or the time allowed for to analyse there too.
And how do you make these takeouts “live” with the management community? Not only is the researcher by definition an “outsider”, do they know how to put them in terms the commercial teams will find interesting and useful? Can they tell a relevant commercial story? Or does the data end up being just a set of declarations about percentages, indices and this-is-bigger-than-that. Unlikely to set the world on fire. And that’s not their fault, it’s a tough ask in the circumstances.
2. Speed
Like most things in life, time is an ingredient to a better outcome. I myself rarely spot all the possible matters on any data side, and I look at them day-in-day-out (maybe that’s its own problem).
More crucially, with a data set as large as ours, the number of permutations is so many that the sheer process of drilling down into the structure to understand cause and effect is inherently time-consuming. Chances are if a summary report is prepared quickly it will miss a lot of nuances and may indeed miss some of the most important outcomes of the research (because these might be “buried” several layers deep). We are greatly helped nowadays by a lot of automation. We can spit out 100s of ppt slides in literally seconds. But that brings a downside. The automation can’t yet tell you which slides are more interesting or useful. You need to review them all. Even if they are nice and pretty, and beautifully colour coded like ours, the more you have the longer the review task.
I’d add one more point. That this isn’t a linear process if done well. The human brain is great at spotting patterns and coming up with ideas. If given the right inputs….and time to think. Ideally, your teams would have time to do an analysis-review-ask cycle more than once. Over many days. If you are to get the real value out. Noting that even the most enthusiastic ppt user among us will get bored and need a break from time to time.
3. Point in time
We talk about Shopper Intelligence as a year-round toolkit, not a research project. But this point applies to any complex research endeavour. As your business goes about its planning and execution questions about the consumer and/or shopper come up all the time. Probably daily. There will be meetings taking place where questions are asked and probably guesses made Assumptions are used as the basis for decisions. What if those assumptions are answered/confirmed in your data, but those questions weren’t in the room when you did the original analysis? Or if the breadth and depth of this data have now been “forgotten”?
A major challenge for insight functions is to keep data sets alive and being used as new issues emerge and more questions are asked. This means creating a “data-oriented” culture and for commercial teams to know about what data is present, what’s available to them to answer their questions.
Better still, “teach them to fish” i.e. get a wider team to be comfortable accessing and using the data directly. The old school debrief and summary of key takeouts (“give them a fish”) detracts from that approach. It implies that the task is finished and the project is now complete. Whereas perhaps it could continue to act as a gold mine for years to come.
The reality is that the best outcomes from research are a collaboration between your researchers and your own people. A 2 + 2 = 5 reality. In summary, perhaps the best question to ask when embarking on a complex and rich research project is from the start “how are we going to maximise the value we get from this data?” not “when can I have the key takeouts?”