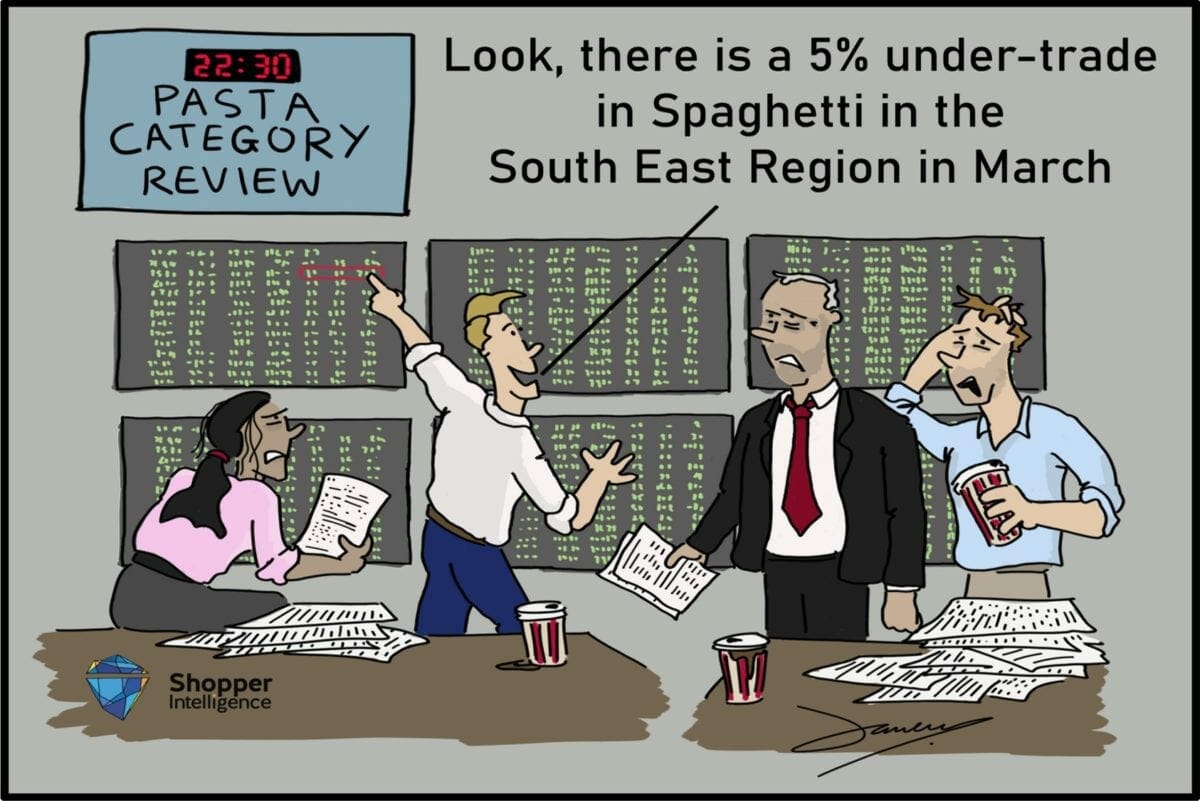
Why isn’t sales history alone sufficient to understand shoppers?
If you read the marketing tomes coming from the likes of Nielsen, IRI, Kantar, 84.51, Dunnhumby etc, you would imagine that historic sales patterns can answer pretty much any category or retail strategy requirement.
There are many providers of sales analytics. An entire Industry. An Industry with a common dominant assumption, an assumption that I think we should be willing to challenge.
Their toolkits give ever more granular analysis on who bought what and when and thus, they say, tell you what you need to do. Now, AI is throwing its oar in the mix and giving solutions that crunch historic sales data in a matter of minutes to give you the recommendation on the promotional plan or the best assortment. The power of algorithms can uncover more detail and new patterns previously impossible to spot or use. Very impressive potential.
I am not going to say these tools are anything other than phenomenally powerful. But I just want to assert that they do have blind spots that limits their utility if we use them as our only input to our thinking.
Can history alone uncover a different future?
The first point here is that, of course, history is predicated on what shoppers have been presented with so far. What they did in the past is driven by what they were offered in the past. To some extent there is a “circularity” in this analysis.
The history could easily lead you to repeat strategies that are under optimized because, put simply, you don’t know any better. History and shoppers can only see what they have seen to date. Historical point of sale data, by definition, cannot measure novel marketing programs or stimuli.
At worst we could be like the Titanic steaming towards an unseen future iceberg, because all the historic evidence gives us no cause for concern.
At best, we risk following a path of ever diminishing returns squeezing a few % point improvements from all our efforts, rather than a step-change that might yield far greater return on investment. I remember a project I worked on where in a particular category the top 10 sellers which had survived years of range reviews because of their individual rate of sale. As it turned out they all met near identical shopper needs and were largely interchangeable. Meanwhile in a different emerging channel a whole new type of product targeting a different need altogether, had emerged but was thus far ignored. Once introduced, category sales saw a step change. Doesn’t happen often, but does happen.
Usually the data is looked at too narrowly
A second problem is that too much analysis in our Industry looks at one category in isolation. At least in part its driven by the pricing model of providers charging on a “per category” basis. But in doing this, we’d be assuming that any category is a closed system, where behavior can be optimized without impacting anything else. A store is far from that. Optimising a store is about using categories as optional “levers”. Getting the best result from the store (best footfall, best basket spend, highest conversion), is about how price, space, merchandising, range is deployed across categories; how categories work in concert or in conflict to meet or stimulate demand.
Walmart specifically stated that a major cause of their sales problems in the 2000s was an overreliance on category specific analytics, but less understanding of how categories combine and work across the store as a whole. Temporarily, sales analytics there were given a back seat. Of course, the pendulum could swing too far the other way, but its crucial that any category is considered in context not in isolation.
We designed Shopper Intelligence to plug this insight “gap” in the view we have of shopper behavior gained from, well let’s say it: “old school” surveys.
The first key addition to existing data comes because our data comes from asking shoppers what they think and want, which gets to the heart of what they truly need. I agree this too may be conditioned by past experience but it’s surprising how insightful it is to overlay the reality of the shopper mindset onto past plans.
For example, historic data would never have led to the creation of a “breakfast biscuit” category. Asking shoppers how and when they ate the biscuits they bought did (I simplify, of course).
Another example: A major retailer delisted a large chunk of ranging in a niche category, because the SKUs didn’t meet “rate of sale benchmarks”. But it turned out that for shoppers buying that category, these “slow sellers” were of such importance that their removal meant shoppers would take their entire basket elsewhere.
Secondly, we present this data as a comparison across all categories (and retailers). There are huge insights from comparing highest to lowest within a department and within a store, not to mention comparing across retailers and channels. We built Shopper Intelligence to put each category firmly in context. One can take a top down “look” or a bottom up “look”. I remember well being part of a category project for a canned soup manufacturer who on discovering 15% of sales were “unplanned”, marched into the retailers to demand and pay for more secondary display. Only to be rejected. The reality was that 15% is one of the lowest levels of unplanned buying for any category in a store. Not such a compelling argument to the retailer, I’d contend. Relativity is crucial.
For us, the perfect analysis should project forward from sales pattern history but then overlay what shoppers want or need more/less of in any category.
That’s particularly important for whole of store/department planning, but its insightful even in comparing segments within a category.
Finally, just a heads up, we are now bringing this same approach and philosophy to in-store observations with our new Store Impact technology – listed amongst the Top 50 retail tech solutions of 2020 in the Discovery 50 list.
About the authors: Roger Jackson is CEO of Shopper Intelligence, with a 30 years of experience in marketing and consumer goods. Bill Bean is a nationally recognized American senior marketing executive with expertise in marketing analytics, shopper insights and innovative tech solutions in shopper research.